Pitfalls and perils of survival analysis under incorrect assumptions: the case of COVID-19 data
English
Abstract
Non-parametric survival analysis has become a very popular statistical method in current medical research. However, resorting to survival analysis when its fundamental assumptions are not fulfilled can severely bias the results. Currently, hundreds of clinical studies are using survival methods to investigate factors potentially associated with the prognosis of coronavirus disease 2019 (COVID-19) and test new preventive and therapeutic strategies.
In the pandemic era, it is more critical than ever to base decision-making on evidence and rely on solid statistical methods, but this is not always the case. Serious methodological errors have been identified in recent seminal studies about COVID-19: One reporting outcomes of patients treated with remdesivir and another one on the epidemiology, clinical course, and outcomes of critically ill patients.
High-quality evidence is essential to inform clinicians about optimal COVID-19 therapies and policymakers about the true effect of preventive measures aiming to tackle the pandemic. Though timely evidence is needed, we should encourage the appropriate application of survival analysis methods and careful peer-review to avoid publishing flawed results, which could affect decision-making.
In this paper, we recapitulate the basic assumptions underlying non-parametric survival analysis and frequent errors in its application and discuss how to handle data on COVID-19.
Downloads
References
Horton NJ, Switzer SS. Statistical methods in the journal. N Engl J Med. 2005;353:1977-9. https://doi.org/10.1056/NEJM200511033531823
Machin D, Campbell MJ, Walters SJ. Medical Statistics: A textbook for the health sciences, 4th edition. Chichester, UK: John Wiley & Sons Ltd.; 2007. p.181-200.
Grein J, Ohmagari N, Shin D, Diaz G, Asperges E, Castagna A, et al. Compassionate use of remdesivir for patients with severe Covid-19. N Engl J Med. 2020;382:2327-36. https://doi.org/10.1056/NEJMoa2007016
Bonovas S, Piovani D. Compassionate use of remdesivir in Covid-19. N Engl J Med 2020;382:e101. https://doi.org/10.1056/NEJMc2015312
Cummings MJ, Baldwin MR, Abrams D, Jacobson BA, Meyer BJ, Balough EM, et al. Epidemiology, clinical course, and outcomes of critically ill adults with COVID-19 in New York City: A prospective cohort study. Lancet. 2020;395:1763-70. https://doi.org/10.1016/S0140-6736(20)31189-2
Piovani D, Bonovas S. Study of critically ill patients with Covid-19 in New York City. Lancet. 2020;396:1064. https://doi.org/10.1016/S0140-6736(20)32072-9
Wolkewitz M, Cooper B, Barnett AG, Binderet N, Schumacher M. Treatment of hospitalacquired pneumonia. Lancet Infect Dis. 2011;11:729. https://doi.org/10.1016/S1473-3099(11)70262-6
Wolkewitz M, Harbarth S, Beyersmann J. Daily chlorhexidine bathing and hospital-acquired infection. N Engl J Med. 2013;368:2330-2. https://doi.org/10.1056/NEJMc1304820
Altman DG, Bland JM. Time to event (survival) data. BMJ. 1997;317:468-9. https://doi.org/10.1136/bmj.317.7156.468
Kaplan EL, Meier P. Nonparametric estimation from incomplete observations. J Am Stat Assoc. 1958;53:457-81. https://doi.org/10.2307/2281868
Bland JM, Altman DG. Statistics notes: Survival probabilities (the Kaplan–Meier method). BMJ. 1998;317:1572. https://doi.org/10.1136/bmj.317.7172.1572
Cox DR. Regression models and life tables. J R Stat Soc B. 1972;34:187-220. https://doi.org/10.1111/j.2517-6161.1972.tb00899.x
Hernan MA. The hazards of hazard ratios. Epidemiology. 2010;21:13-5. https://doi.org/10.1097/EDE.0b013e3181c1ea43
Rulli E, Ghilotti F, Biagioli E, Porcu L, Marabese M, D’Incalci M, et al. Assessment of proportional hazard assumption in aggregate data: A systematic review on statistical methodology in clinical trials using time-to-event endpoint. Br J Cancer. 2018;119:1456-63. https://doi.org/10.1038/s41416-018-0302-8
Royston P, Parmar MK. Flexible proportional-hazards and proportional-odds models for censored survival data, with application to prognostic modelling and estimation of treatment effects. Stat Med. 2002;21:2175-97. https://doi.org/10.1002/sim.1203
Wolkewitz M, Cooper BS, Bonten MJ, Barnett AG, Schumacher M. Interpreting and comparing risks in the presence of competing events. BMJ. 2014;349:g5060. https://doi.org/10.1136/bmj.g5060
Lau B, Cole SR, Gange SJ. Competing risk regression models for epidemiologic data. Am J Epidemiol. 2009;170:244-56. https://doi.org/10.1093/aje/kwp107
Southern DA, Faris PD, Brant R, Galbraith PD, Norris CM, Knudtson ML, et al. Kaplan-Meier methods yielded misleading results in competing risk scenarios. J Clin Epidemiol. 2006;59:1110-4. https://doi.org/10.1016/j.jclinepi.2006.07.002
Austin PC, Lee DS, Fine JP. Introduction to the analysis of survival data in the presence of competing risks. Circulation. 2016;133:601-9. https://doi.org/10.1161/CIRCULATIONAHA.115.017719
Schuster NA, Hoogendijk EO, Kok AAL, Twisk JWR, Heymans MW. Ignoring competing events in the analysis of survival data may lead to biased results: A nonmathematical illustration of competing risk analysis. J Clin Epidemiol. 2020;122:42-8. https://doi.org/10.1016/j.jclinepi.2020.03.004
Austin PC, Fine JP. Accounting for competing risks in randomized controlled trials: A review and recommendations for improvement. Stat Med. 2017;36:1203-9. https://doi.org/10.1002/sim.7215
Fine J, Gray RJ. A proportional hazards model for the subdistribution of a competing risk. J Am Stat Assoc. 1999;94:496-509. https://doi.org/10.1080/01621459.1999.10474144
Berry SD, Ngo L, Samelson EJ, Kiel DP. Competing risk of death: An important consideration in studies of older adults. J Am Geriatr Soc. 2010;58:783-7. https://doi.org/10.1111/j.1532-5415.2010.02767.x
Wolkewitz M, Sommer H. Evaluating mortality in an intensive care unit requires extended survival models. Crit Care. 2014;18:415. https://doi.org/10.1186/cc13757
Wang Y, Zhang D, Du G, Du R, Zhao J, Jinet Y, et al. Remdesivir in adults with severe Covid-19: A randomised; double-blind, placebo-controlled, multicentre trial. Lancet. 020;395:1569-78. https://doi.org/10.1016/S0140-6736(20)31022-9
Beigel JH, Tomashek KM, Dodd LE, Mehta AK, Zingman BS, Kalil AC, et al. Remdesivir for the treatment of Covid-19 - Final Report. N Engl J Med. 2020;383:1813-26. https://doi.org/10.1056/NEJMoa2007764
The RECOVERY Collaborative Group. Dexamethasone in hospitalized patients with Covid-19 -Preliminary Report. N Engl J Med. 2021;384:693-704. https://doi.org/10.1056/NEJMoa2021436
The RECOVERY Collaborative Group. Effect of hydroxychloroquine in hospitalized patients with Covid-19. N Engl J Med 2020;383:2030-40. https://doi.org/10.1056/NEJMoa2022926
The RECOVERY Collaborative Group. Lopinavir-ritonavir in patients admitted to hospital with COVID-19 (RECOVERY): A randomised, controlled, open-label, platform trial. Lancet. 2020;396:1345-52. https://doi.org/10.1016/S0140-6736(20)32013-4
Piovani D, Pansieri C, Peyrin-Biroulet L, Danese S, Bonovas S. A snapshot of the ongoing clinical research on COVID-19. F1000Res 2020;9:373. https://doi.org/10.12688/f1000research.23843.1
Janiaud P, Axfors C, Van’t Hooft J, Saccilotto R, Agarwal A, Appenzeller-Herzog C, et al. The worldwide clinical trial research response to the COVID-19 pandemic – the first 100 days. F1000Res 2020;9:1193. https://doi.org/10.12688/f1000research.26707.2
Some similar items:
- José Moreno-Montoya, The challenge of communicating and controlling the coronavirus epidemic , Biomedica: Vol. 40 No. 1 (2020)
- Juan Pimentel, Neil Andersson, Chloroquine and its derivatives in the management of COVID-19: A scoping review , Biomedica: Vol. 40 No. Supl. 2 (2020): SARS-CoV-2 y COVID-19
- Lida Carolina Lesmes-Rodríguez, Luz Natalia Pedraza-Castillo, Dumar Alexander Jaramillo-Hernández, HCoV-NL63 and HCoV-HKU1 seroprevalence and its relationship with the clinical features of COVID-19 patients from Villavicencio, Colombia , Biomedica: Vol. 44 No. 3 (2024)
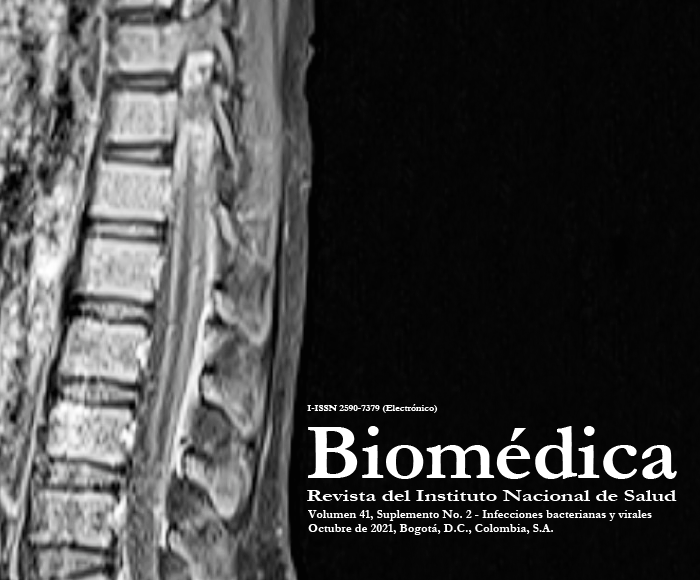
Article metrics | |
---|---|
Abstract views | |
Galley vies | |
PDF Views | |
HTML views | |
Other views |